Teaching
We conduct research on the intersection between digital entrepeneurship, digital ecosystems, and organizing data and knowldedge. We will provide multiple courses that help to explain changes on firm- and ecosystem levels using multiple theories and methodologies. Our teaching is usually highly interactive and builds upon concepts of blended learning, oftentimes 'flipping the classroom'. It is important to us to not only reflect latest findings of information systems research with our students but also to work together on applying that knowledge.
Summer '24
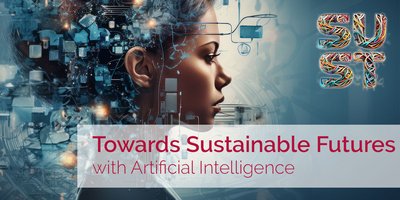
Lecture & Tutorial
Towards Sustainable Futures with AI
- Lecturer:
- Prof. Dr. Hannes Rothe
- Mahnoor Shahid, M.Sc.
- Contact:
- Term:
- Summer Semester 2024
- Time:
- Fridays, 10:00 - 14:00
- Room:
- R09 R04 H02
- Start:
- 12.04.2024
- End:
- 14.07.2023
- Language:
- English
Description:
Artificial Intelligence (AI) is widely considered a generative technology that has the potential to have great impact on our society, economy, and ecology. Whether these impacts will be for worse or for better is up for discussion and depends on the actions of individuals, companies, and authorities worldwide towards the 18 UN Sustainable Development Goals.
Throughout the lecture series, students get familiar with concepts and theories that describe and explain AI companies, and learn about the design of Machine Learning-based applications. Do we need AI – or does AI solve our problems? What problems can machine learning effectively solve? What is the current impact of AI technologies on economy, society and ecology? How can we apply AI to a new domain or problem? What role do humans play in designing AI applications?
Building on fundamentals of information systems strategy and enterprise modelling, students reflect the impact of strategy and organizing in AI companies towards their ability to produce sustainable futures. We particularly investigate the generative capacity of data, tools, and (machine learning) models to produce such futures. Among others, we will cover the impact of biases in data and algorithms, explainability of AI applications, as well as accuracy, sovereignty, (inverse) scalability and framing of ML models. Throughout the entire module, we critically reflect impacts of managerial and algorithmic decision-making on sustainability, this includes impacts, for instance, on aspects of health and well-being (SDG 3), gender equality (SDG 5), or climate action (SDG 13).
Learning Targets:
Students will be able to
|
Outline:
• AI Companies & Data-centric Thinking
• Sustainable Information Systems
• Strategy & AI Companies for Sustainable Futures
• Organization & AI Companies for Sustainable Futures
• Managing Machine Learning Projects for Sustainable Futures
• Building AI Applications
• Generativity and Boundaries from Digital Tools
• Generativity and Boundaries from Data
• Generativity and Boundaries from (ML) Models
Literature:
- Berente, N., Gu, B., Recker, J., & Santhanam, R. (2021). Managing artificial intelligence. MIS Quarterly, 45(3).
- Brynjolfsson, E., & Mcafee, A. (2017). Artificial intelligence, for real. Harvard Business Review, 1, 1-31.
- Brynjolfsson, E., Rock, D., & Syverson, C. (2018). Artificial intelligence and the modern productivity paradox: A clash of expectations and statistics. In The economics of artificial intelligence: An agenda (pp. 23-57). University of Chicago Press.
- Fürstenau, D., Baiyere, A., Schewina, K., Schulte-Althoff, M., and Rothe, H. (forthcoming). Extended Generativity Theory on Digital Platforms, Information Systems Research.
- Gregory, R. W., Henfridsson, O., Kaganer, E., & Kyriakou, H. (2021). The role of artificial intelligence and data network effects for creating user value. Academy of Management Review, 46(3), 534-551.
- Provost, F., & Fawcett, T. (2013). Data Science for Business: What you need to know about data mining and data-analytic thinking. O'Reilly Media, Inc.
- Raisch, S., & Krakowski, S. (2021). Artificial intelligence and management: The automation–augmentation paradox. Academy of Management Review, 46(1), 192-210.
- Russell, S., & Norvig, P. (2021). Artificial Intelligence, Global Edition: A Modern Approach. (4th ed.). Pearson Education.
Further literature will be provided during the course